Cardiac Risk Toolset
Toolset description
This study was designed to improve the cardiovascular risk assessment, in particular coronary heart disease and heart failure patients, as a tool to support the clinical decision. The main goal is to incorporate, in a flexible and personalized way, data/knowledge from several sources of information. To this aim, a proper combination scheme was developed, able to combine in a global tool well-known current risk assessment tools as well as physician’s clinical knowledge.
In a first phase, all the relevant CVD risk assessment tools used in current clinical practice, which are described through different methods (equations, scores, etc.), were represented based on a common description – Bayesian classifiers. In a second phase, the combination of individual models was performed through the fusion of the individual models’ parameters (conditional probabilities). The resultant parameters were adjusted based on an optimization procedure, carried out with genetic algorithms (GA).
The validation was performed in the context of the secondary prevention. GRACE, TIMI and PURSUIT were the selected risk assessment tools to be combined. A real patient dataset, Santa Cruz Hospital, Lisbon/Portugal, N=460 Acute Coronary Syndrome with non-ST segment elevation (ACS-NSTEMI) patients was considered in this validation procedure.
The software allows the input of risk factors of a specific patient, calculates the respective CVD risk, and shows it according to the two defined categories: {“low risk”, “high risk”}. The physician can easily assess the risk of a specific patient or he can use the tool as a predictor, in the sense that can input different values for several parameters and foreseen the result of its adjustment.
The physician can assess the risk of an individual patient without any additional configuration of the model (default parametrization) or, on the contrary, configure the Bayesian global model. Thus, the physician may load a previously optimized parameters for the global model or alternatively perform a set of new configurations that are going to originate new values for the parameters of the global model. The physician also has the possibility of selecting the risk factors that are going to integrate the global model as well as adjust the weights of the individual models. Additionally, this configuration includes an optimization procedure (GA approach) to calibrate the global model to a specific population.
Input data are organized according to six categories:
Demographics |
|||
1 |
Age |
Discrete |
years; [40, 90] |
2 |
sex |
Boolean |
0/1 – Female/Male |
History |
|||
3 |
Risk Factors > 3 |
Boolean |
diabetes, smoking, family history, hypertension, hypercholesterol |
4 |
Aspirin |
Boolean |
No/Yes |
5 |
known CAD |
Boolean |
No/Yes |
6 |
Angina |
Boolean |
No/Yes |
Clinical |
|||
7 |
Cardiac Arrest at Admission |
Boolean |
No/Yes |
8 |
Elevated Cardiac Markers |
Boolean |
No/Yes |
9 |
CCS > II (angina classification) |
Boolean |
No/Yes |
10 |
HF signs (heart failure) |
Boolean |
No/Yes |
11 |
ERL (enrollment) |
Boolean |
No/Yes |
12 |
Killip |
Ordinal |
{1, 2, 3, 4} |
Laboratory |
|||
13 |
Cr (Creatinine) |
Continuous |
[ 0, 40] |
Measurements |
|||
14 |
SBP (systolic blood pressure) |
Continuous |
[40, 250] |
15 |
HR (heart rate) |
Continuous |
[10, 200] |
ECG |
|||
16 |
STD (ST segment deviation) |
Boolean |
No/Yes |
Data characteristics
The data used contains information from 460 consecutive patients admitted with NSTE-ACS between March 1999 and July 2001. The event rate of the combined endpoint (death/myocardial infarction) was 7.2% (33 events).
The characteristics of the data are provided in the Table below.
Model | Event |
Age (years) | 63.4 ± 10.8 |
Sex (Male/Female) | 361 (78.5%) / 99 (21.5%) |
Risk Factors: Diabetes (0/1) Hypercholesterolemia (0/1) Hypertension (0/1) Smoking (0/1) |
352 (76.5%) / 108 (23.5%) 180 (39.1%) / 280 (60.9%) 176 (38.3%) / 284 (61.7%) 362 (78.7 %) / 98 (21.3%) |
Previous History / Known CAD: Myocardial Infarction (0/1) Myocardial Revascularization (0/1) PTCA CABG |
249 (54.0%) / 211 (46.0%) 239 (51.9%) / 221 (48.1%) 146 (31.7%) 103 (22.4%) |
Sbp (mmHg) | 142.4 ± 26.9 |
Hr (bpm) | 75.3 ± 18.1 |
Creatinine (mg/dl) | 1.37 ± 1.26 |
Enrolment [0 UA, 1 MI] | 180 (39.1 %) / 280 (60.9%) |
Killip 1/2/3/4 | 395 (85.9%) / 31 (6.8%) / 33 (7.3 %) / 0% |
CCS [0 I/II; 1 CSS III/IV] | 110 (24.0%) / 350 (76.0%) |
ST Segment Deviation (0/1) | 216 (47.0%) / 244 (53.0%) |
Signs of Heart Failure(0/1) | 395 (85.9%) / 65 (14.1%) |
Tn I > 0.1 ng/ml (0/1) | 313 (68.0%) / 147 (32.0%) |
Cardiac Arrest Admission (0/1) | 460 (100%) / 0% |
Aspirin (0/1) | 184 (40.0%) / 276 (60.0%) |
Angina (0/1) | 19 (4.0%) / 441 (96.0%) |
CABG: coronary artery bypass grafting; CAD: coronary artery disease; CCS: Canadian Cardiovascular Society angina classification; MI: myocardial infarction; n: no; PTCA: percutaneous transluminal coronary angioplasty; SBP: systolic blood pressure; TnI: troponin I; UA: unstable angina; y: yes.
Notes: Continuous variables with a normal distribution are expressed as mean value and standard deviation. Discrete variables are presented as frequencies and per cent values.
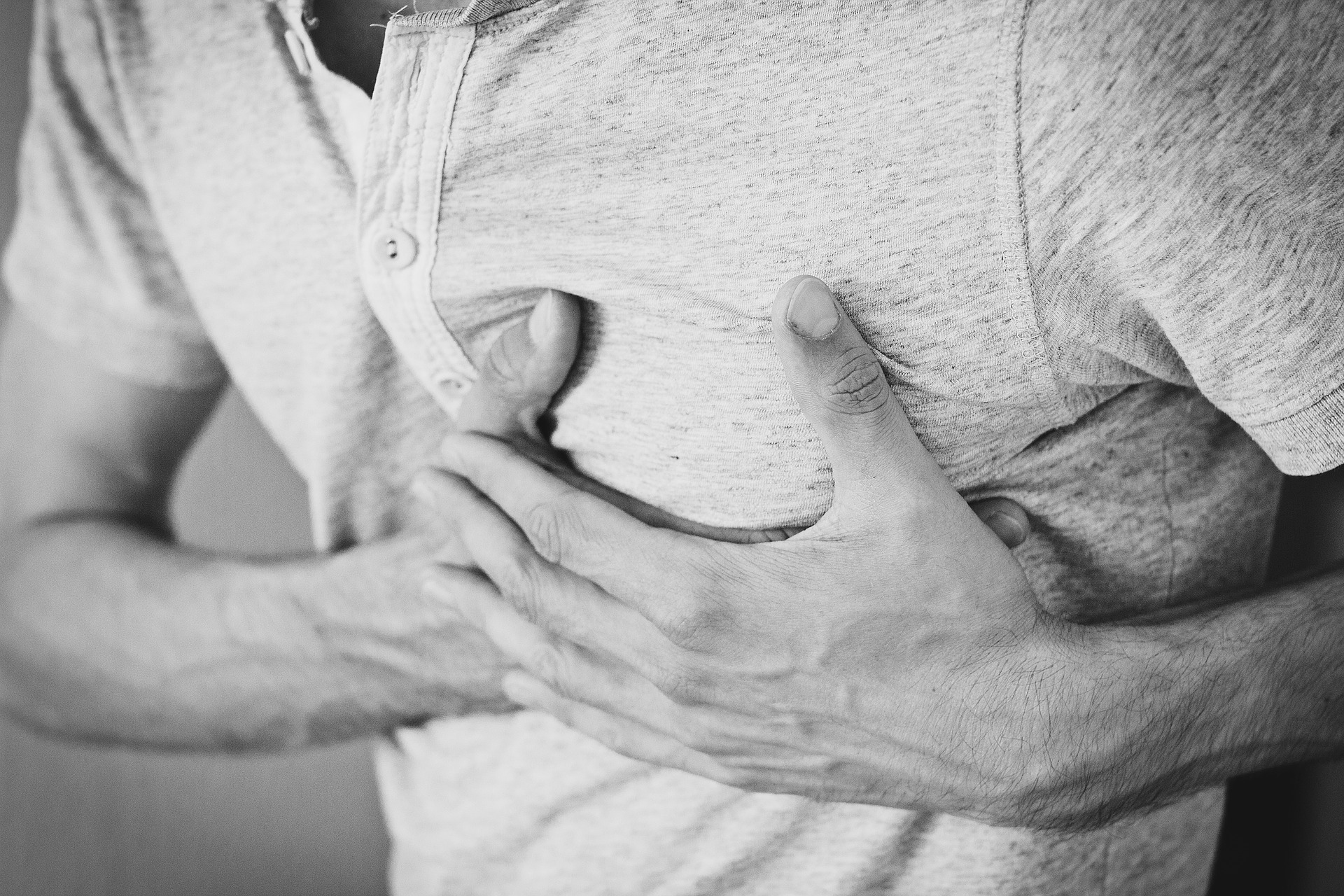
Access:
This dataset is not publicly available. If you are interested in this problem and in the toolset please contact us. The access will be analysed case by case.
How to cite this database:
Paredes, S., Rocha, T., Mendes, D., Carvalho, P., Henriques, J., Morais, J., Ferreira, J. and Mendes, M., 2016. New approaches for improving cardiovascular risk assessment. Revista Portuguesa de Cardiologia, 35(1), pp.5-13.